
- Products
- Solutions
- Learn
- Partner
- Try Now
Natural Language Processing is becoming more important in the field of Human-Computer Interaction. This paper addresses a search interface to convert Natural Language Query (example English Language) into the database system understandable language without having knowledge of system language. The main goal of this system is to provide communication between human and computer without recalling any sort of database DDL or DML query syntax. The more general goal of such search interface or NLP system is to make the computer able to understand the natural language so that user can address the system as if they are addressing some other person and get the expected result they want.
NLQ is one of the most useful AI disciplines, however, we’ve recently started finding out about it related to BI(Business intelligence) and data analytics. In this blog, I would like to explore more about NLQ and talk about how this AI innovation can be utilized in our space.
NLQ serves those users who want quick results or lack the talents or permission to model their data visualization tools or code editors. Therefore, if we predict concerning information access as a operate of technical skills and also the time it takes to urge a solution, NLQ is the primary technology that users can communicate once looking to find insights.
There are typically two possible goals that drive the first inquiry a client asks when entering a framework or application. Take Spotify, for example. At the point when the application is first opened, the client might look for a particular song that was heard while passing by the neighborhood cafe or the client might need to be amazed at, suppose, a song from the new trial collection by a Yemen Reggae folk artist. NLQ can serve each of those experiences using an analytic moment or an exploration mode. preferably, the NLQ engine will locate the motive of the user, input into any such experiences based on the primary query, and then facilitate the path accordingly.
In a fraction of the analytic second, the person is focused on finding the answer to a specific query. The NLQ capabilities on an ‘ask a selected query, get a selected answer’ system. we discover, in popular, that greater than 60% of the searches are round “analytic moments,” or ad-hoc searches, based normally through OEM consumption and APIs.
On the alternative end of the NLQ experience, we have exploration mode. In this mode, we discover that the client has a vague idea of what they want to discover. Generally, in this mode, the person runs a searching a general subject, saves the intermediate effects, and splits those effects into some different guidelines. Let’s pass back to our Spotify instance. The client will start off with a wide search topic like “folk tune” as an example. The consequences will come lower back with folks music from around the world, and without a unique route in mind, the person will start to drill down into international locations, then local streams of a hobby, and subsequently land on a Yemen reggae folks artist. In this mode, the user avoids putting too much effort into the definition of a selected seek, and rather, is based on a random exploration path with the assisted exploration of NLQ.
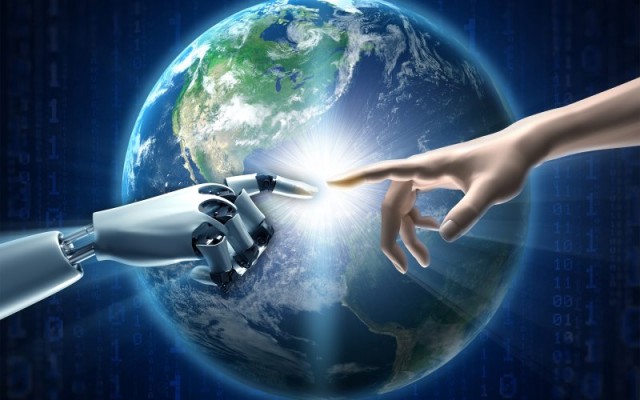
Machine Intent vs. User Intent
On the alternative end of the NLQ experience, we have exploration mode. In this mode, we discover that the client has a vague idea of what they want to discover. Generally, in this mode, the person runs a searching a general subject, saves the intermediate effects, and splits those effects into some different guidelines. Let’s pass back to our Spotify instance. The client will start off with a wide search topic like “folk tune” as an example. The consequences will come lower back with folks music from around the world, and without a unique route in mind, the person will start to drill down into international locations, then local streams of a hobby, and subsequently land on a Yemen reggae folks artist. In this mode, the user avoids putting too much effort into the definition of a selected seek, and rather, is based on a random exploration path with the assisted exploration of NLQ.
What if the NLQ engine mistakenly took the query above to intend “how many customers are responsible for 80% of my Q1 2018 income compared to 2017?” when the user actually meant to compare between Q1 2018 to the whole of 2017?
What we need here is the capability to edit the intent detected by using NLQ and update it with the reason of the consumer. This is called “Active Learning,” and it allows in a quicker optimization curve for a greater personalized query, which through the years will become an inherent part of the NLQ.
The usage of AI to its fullest
NLQ is gaining traction within the big data analytics tools area for its brief answers and simplicity of use. By using two very distinct experiences, ‘Analytic moment and Exploration mode’, NLQ accurately serves a huge range of queries and skillsets making it the go-to AI era for plenty of analysts and commercial enterprise users. As for beginning NLQ in analytics, even though there are still challenges with historical data, there are answers that can assist.
There are many activities taking place with Artificial Intelligence today, from experimental to real use instances. In our AI group, we’re making principal towards the destiny of AI by being able to tie the lineage, from ingestion through coaching to analytics, all on metadata (and to semantics inside the future), integrating AI into every component of the ‘Business Intelligence Lifecycle’. I’m fortunate to be part of this rapid-paced AI exploration and to look at how our clients are the use of our developments!
If you enjoyed reading this, we’re sure you will also love checking out what we have in store on our Youtube channel. You can also head to our home page for more info!
Subscribe to stay ahead with the latest updates and entrepreneurial insights!
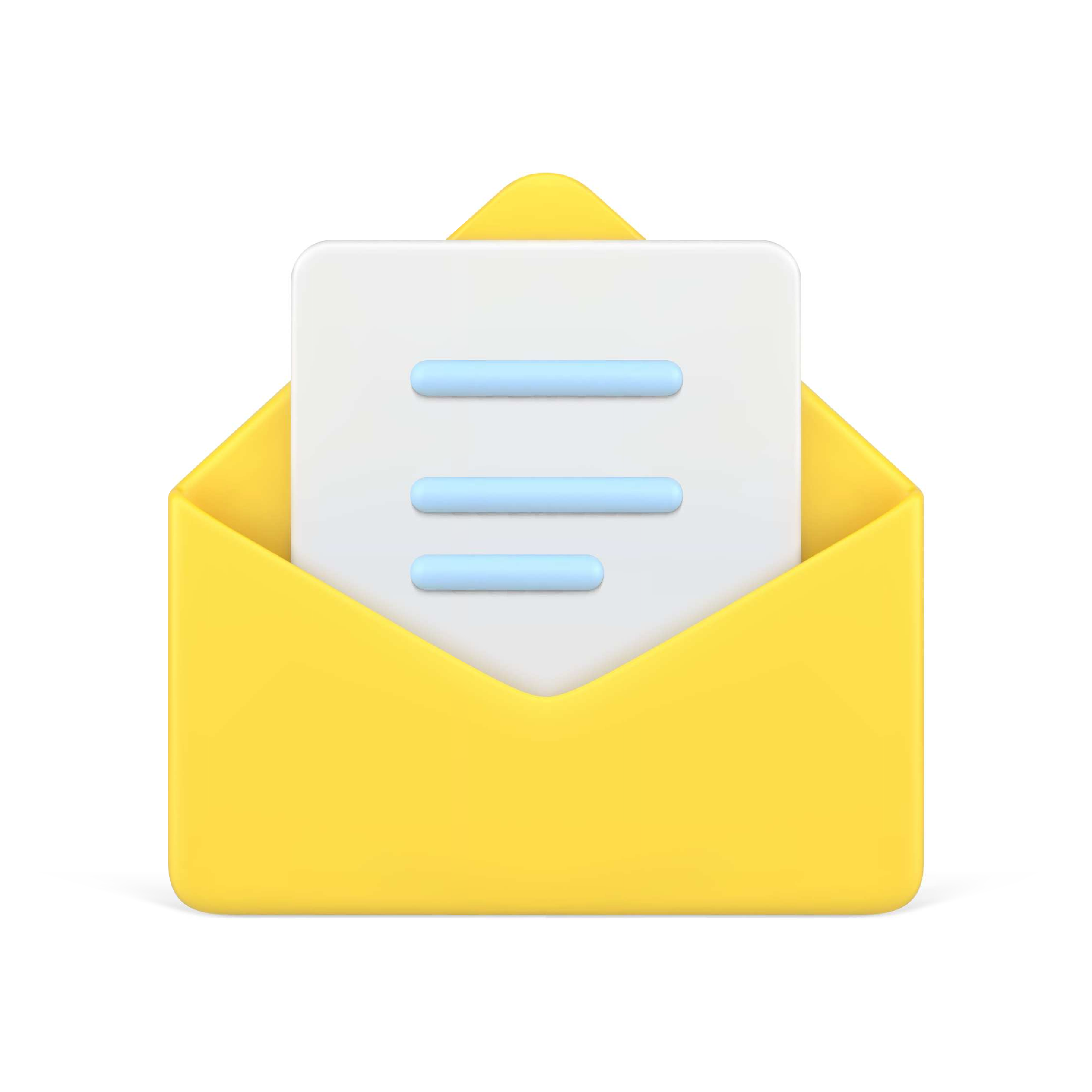
Subscribe to our newsletter
Get access to the latest industry & product insights.